- datapro.news
- Posts
- ETL in the Age of AI
ETL in the Age of AI
THIS WEEK: The consequences hidden bias in data sets when using neural networks
Dear Data Professional…
One of the mainstay processes of Data Engineering is ETL (Extract, Transform, Load). Something that is typically used with Data Warehouses and in Analytics each and every day. This week we thought we’d highlight how AI is transforming traditional ETL processes.
10 ways Generative AI is revolutionising ETL processes:
1. Enhanced Data Extraction: AI technologies are expanding the scope of data extraction by identifying and pulling relevant information from a wider array of sources, including unstructured data like emails and social media posts. This allows for more comprehensive data collection and analysis.
2. Intelligent Data Transformation: Machine learning and AI algorithms are automating complex data cleaning and preparation tasks. They can predict and correct errors, fill in missing values, and identify anomalies in the data, leading to more accurate and reliable transformations.
3. Adaptive Learning: Machine learning algorithms continuously improve their performance as they process more data. This means the ETL process becomes more efficient and effective over time, adapting to new patterns and trends in the data.
4. Optimized Data Loading: AI helps optimize how data is stored and accessed, ensuring that the most relevant information is readily available for analysis. It can also assist in categorizing and tagging data, making it easier to retrieve and use across various business functions.
5. Automation of Routine Tasks: AI-driven ETL processes can automate many routine tasks that previously required manual intervention. This frees up data engineers and analysts to focus on more strategic aspects of data management and analysis.
Like what you’re reading and think someone you know could benefit?
6. Improved Scalability: AI-powered ETL solutions are better equipped to handle large volumes of data and can scale more easily as data sources and volumes grow.
7. Real-time Processing: AI enables near real-time processing of data, allowing organisations to respond more quickly to changing data trends and patterns.
8. Enhanced Data Quality: AI-driven ETL processes incorporate advanced data quality assurance mechanisms, including automatic data validation and error logging, ensuring higher data integrity throughout the ETL pipeline.
9. Intelligent Error Handling: AI can predict and prevent potential errors in the ETL process, as well as automatically resolve issues that may arise, reducing downtime and improving overall reliability.
10. Cross-platform Integration: AI facilitates better integration of ETL processes across diverse environments, enabling more seamless data flow between different platforms and systems.
By leveraging AI for ETL processes, organisations can achieve faster, more accurate, and more insightful data integration. This transformation not only streamlines data management but also enables businesses to derive more value from their data assets, supporting better decision-making and driving digital innovation.
Your Brilliant Business Idea Just Got a New Best Friend
Got a business idea? Any idea? We're not picky. Big, small, "I thought of this in the shower" type stuff–we want it all. Whether you're dreaming of building an empire or just figuring out how to stop shuffling spreadsheets, we're here for it.
Our AI Ideas Generator asks you 3 questions and emails you a custom-built report of AI-powered solutions unique to your business.
Imagine having a hyper-intelligent, never-sleeps, doesn't-need-coffee AI solutions machine at your beck and call. That's our AI Ideas Generator. It takes your business conundrum, shakes it up with some LLM magic and–voila!--emails you a bespoke report of AI-powered solutions.
Outsmart, Outpace, Outdo: Whether you're aiming to leapfrog the competition or just be best-in-class in your industry, our custom AI solutions have you covered.
Ready to turn your business into the talk of the town (or at least the water cooler)? Let's get cracking! (And yes, it’s free!)
STORY: The unintended consequences of hidden bias in training data sets
The neural networks used for Generative AI relies heavily on quality training data sets. The old adage of GIGO (Garbage In, Garbage Out) takes on a new importance when using a neural network to draw inferences from information. There are no records to be deleted in the context of a Large Language Model. To prevent aberrant answers where poor data quality is the cause, your choices can boil down to re-training, or to put guard-rails in place. This weeks story looks at how the misidentification of images in the digital realm, can be traced back to the unconscious basis reflected in analogue images dating back over a century.
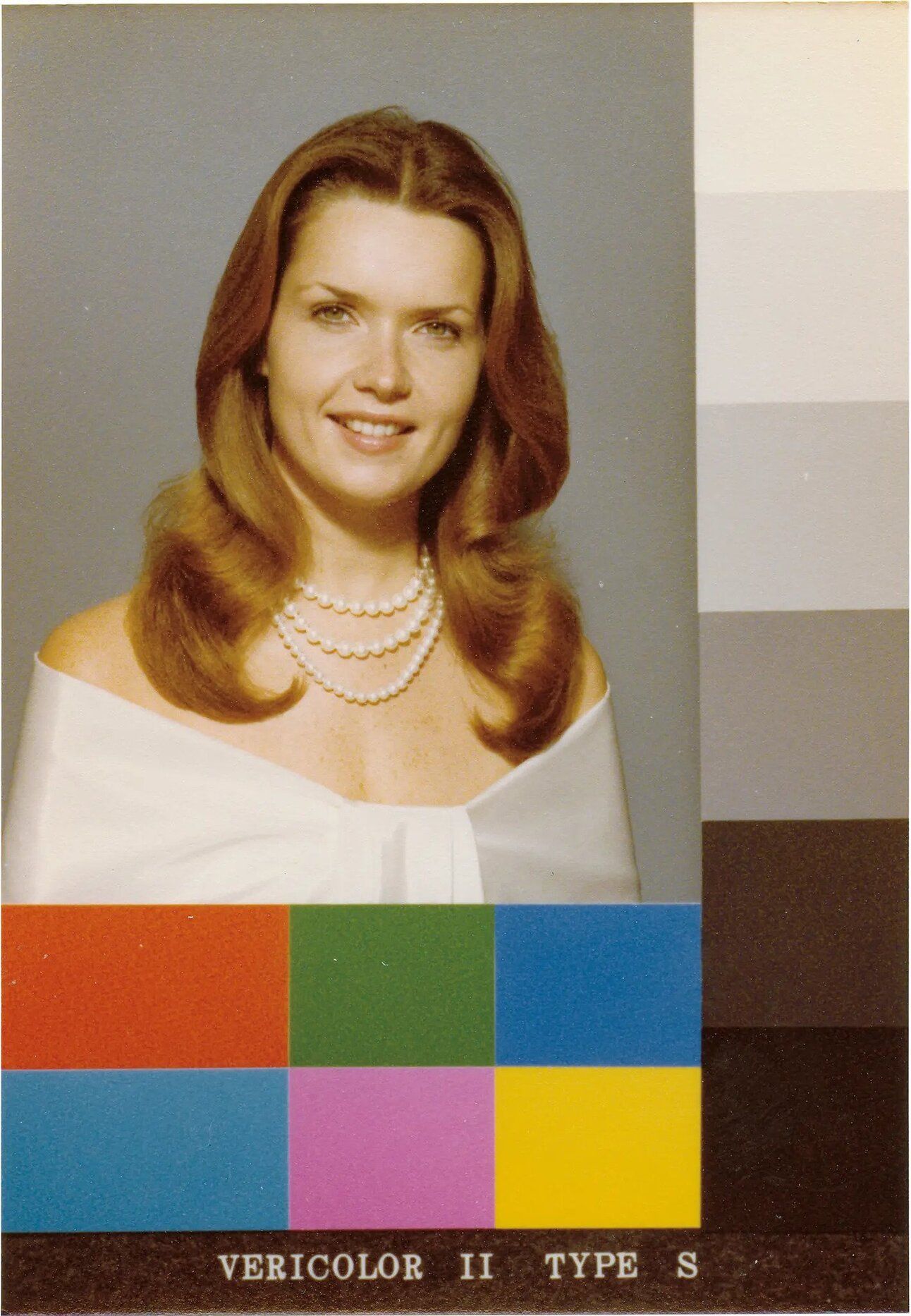
Kodak Shirley card (1978) courtesy of Hermann Zschiegner
In the film developing process the Kodak Shirley Card was used to calibrate the colours cyan, yellow, and magenta for output images. In the field of digital image recognition the choice of a white woman as the model for the card has had lasting impacts on the representation of darker skin tones. This extended into the data sets used to train neural networks and AI technologies for the purpose of image recognition. Because the Shirley card was used to set the standard for colour balance in photo development the calibration process was optimised for lighter skin tones. This led to poor reproduction of darker skin tones. Photos of people with darker skin often appeared underexposed, with a loss of detail and contrast, making them look unnaturally dark and even unrecognisable to a neural network. Some of you may recall the 2015 controversy surrounding the misidentification of images of Black people as primates.
It turns out that this phenomenon can be traced back to the use of the Shirley Card and the hidden bias that was a consequence of calibrating film photography to white skin tones. The lost contrast and detail mean’t that the data sets used to train the Google image recognition neural network were faulty. Interestingly enough the fix implemented was to switch off the network’s ability to recognise primates, the New York Times reported in 2023. It seems that the training data set was so misaligned a fix had not been implemented some 8 years on.
The bias from the Shirley card era carried over into the datasets used to train modern neural networks. These datasets often lacked sufficient representation of darker skin tones, leading to AI systems that perform poorly on images of people with darker skin. The algorithms have been found to falsely identify Black and Asian faces 10 to 100 times more often than white faces, and these errors are more pronounced for women, particularly Black women.
The lessons contained in the hidden bias of Shirley Cards are applicable in Data Integration processes, Data Governance and Data Quality practices. This is especially relevant as we come to be using more and more synthetically generated data for testing and training. The legacy of the Kodak Shirley Card highlights the importance of diverse and representative training data used to train LLM’s.
That’s a wrap for this week.
For more on these topics check out the Data Radio Show - on YouTube and where you get your podcasts.